Dates: 26-29 February 2024
Place: Västerås, Sweden
The programme of the 2024 Winter School can be downloaded from here.
The 2024 winter school is organised with the participation of Ericsson, Saidot, Volvo and RISE.
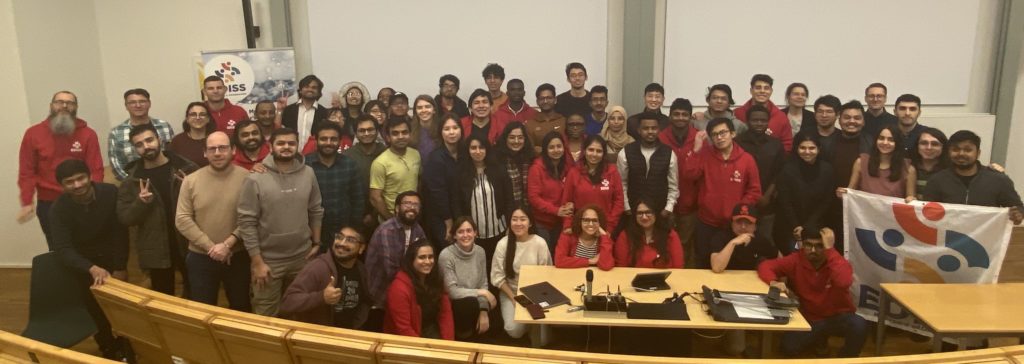
Student posters and videos
Team Apnea
Prediction
Mariama Serafim de Oliveira, Halidu Abdulai, Khizar Muzaffar, Thinakone Louangdy
Apnea is a common condition in premature babies that can negatively affect their neurodevelopment. The aim of this study is to predict and forecast apnea in preterm babies using the Edi signal.
We developed two custom deep learning architectures and employed three classical machine learning algorithms (Logistic Regression, Random Forest, and XGBoost) to detect apnea using a supervised approach. Our preliminary results indicate that the deep learning approach can achieve an accuracy of over 95% in apnea detection, which exceeds the results of previous studies.
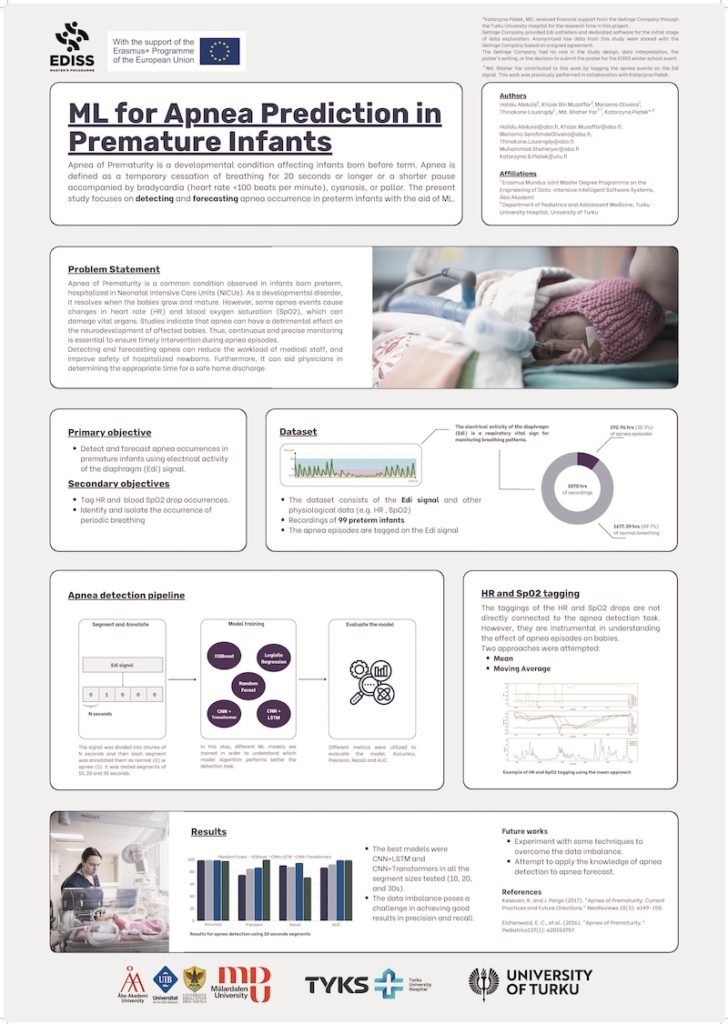
TEAM
XAI Texture Analysis
Pragati Manandhar, Md Masum Billah, Alejandro Cedillo, Sarosh Krishan
Our innovative research takes a fresh look at how AI can help diagnose cancer more clearly. We’re using smart techniques to pick out and study patterns in medical images, which helps us understand how AI thinks. Our approach uses well-designed AI models to identify these patterns accurately, shedding light on the AI’s decision-making. This important work is about making AI in healthcare more transparent and trustworthy, leading to better tools for doctors and safer care for patients.
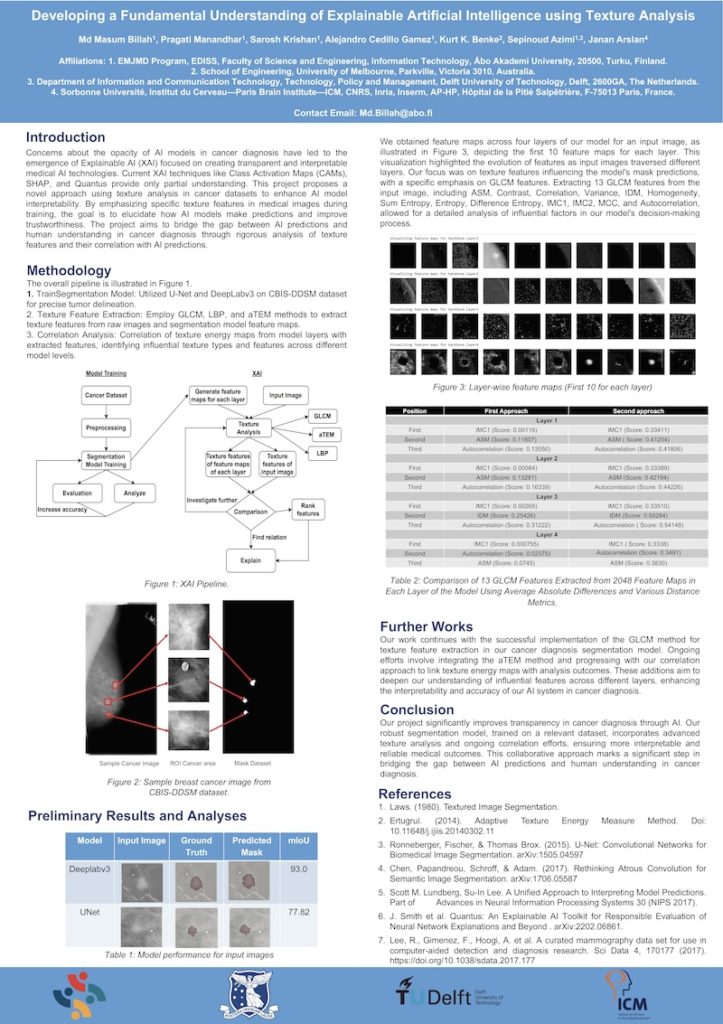
Team Parkinson
Alaina Faisal, Umar Faruk Abdullahi, Ali Kaya, Ammara Asif, Joaquin Caballero
Parkinson’s disease (PD) is a complex neurological disorder characterized by motor symptoms such as tremors and stiffness, alongside non-motor issues, predominantly affecting the substantia nigra region of the brain. Despite extensive research, its underlying molecular mechanisms remain elusive. To bridge this gap, our research harnesses omics data and employs advanced deep neural network techniques like Multilayer Perceptron and Convolutional Neural Network. Through this approach, we achieve an accuracy of over 82% in PD positive detection.
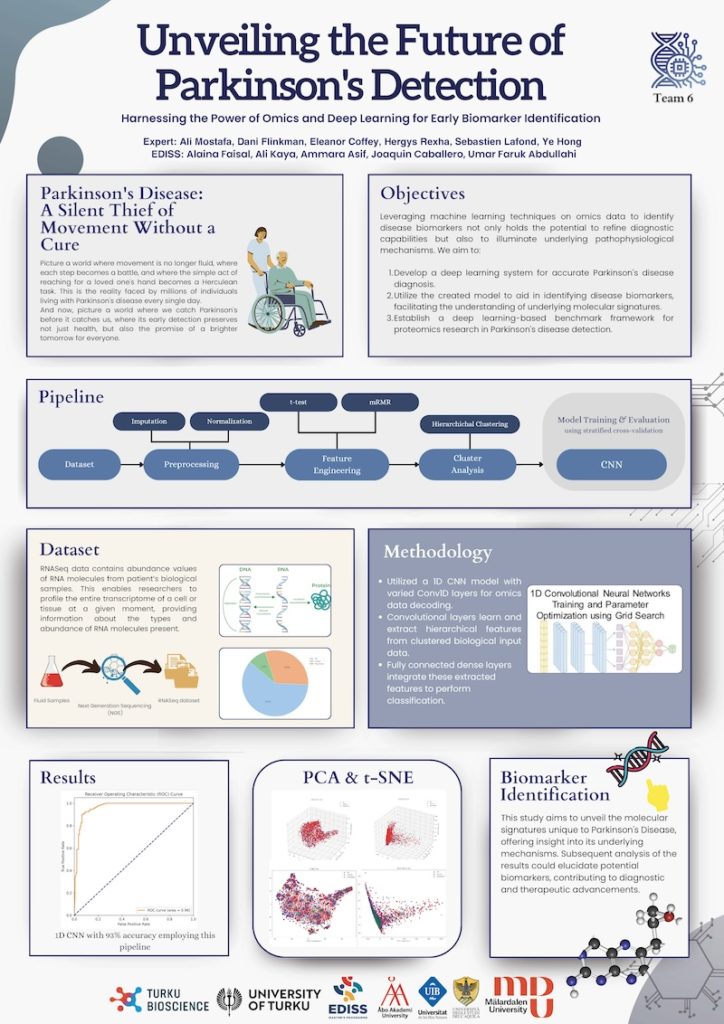
Team Gearbox
Puja Dhakal, Harry Hamjaya, Shihabur Samrat, Motunrayo Ibiyo
Our research introduces an automated system for gearbox manufacturing quality control, leveraging sound analysis to achieve 88% anomaly detection accuracy, notably through the OC-SVM algorithm’s precise semi-supervised learning.
This innovation reduces dependence on manual checks and proposes a strategy for seamless industrial integration, markedly improving quality control practices.
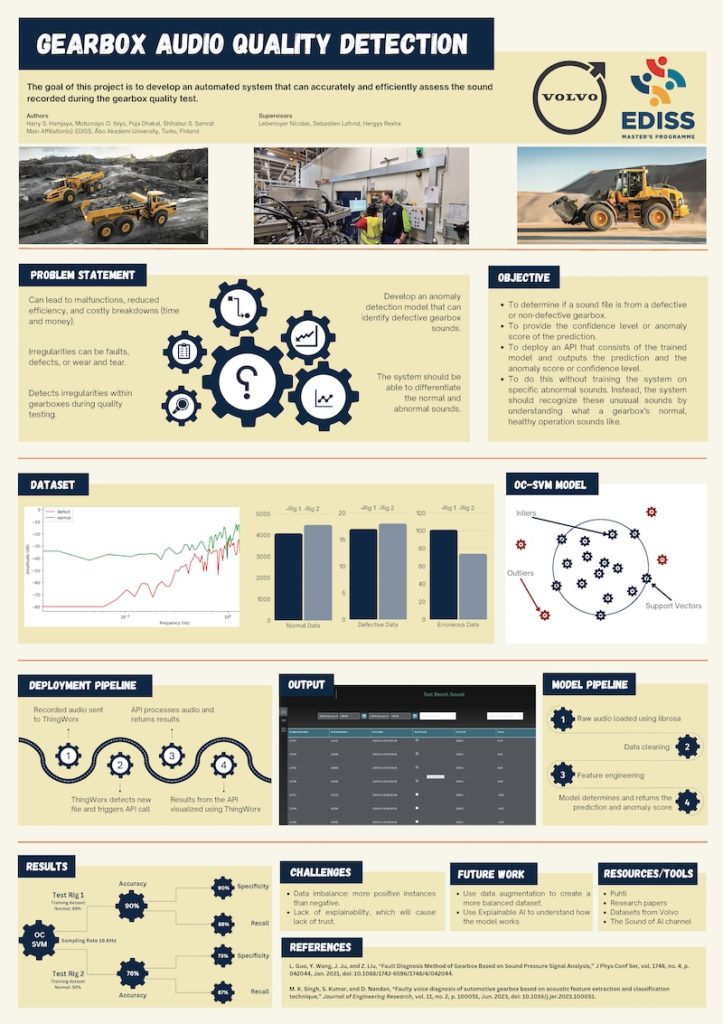
TEAM Configurations in Critical Adaptive Distributed Embedded Systems
Vinay Sanga, Ahmad Kamal Baig, Somoy Barua, Mira Budenova
A Distributed Embedded System (DES) is a network of nodes performing cooperative tasks to meet a common objective.
They are crucial for applications needing high reliability and must sustain their operations even when a part of the system node fails.
We implement a cutting-edge Deep Reinforcement Learning Technique to train an agent that can find suitable configurations for these systems. Our model of Approach (LSTM-PPO with Curriculum Learning and Action Masking) can successfully allocate tasks in fault-tolerant way by replicating critical tasks and allocating them in separate nodes
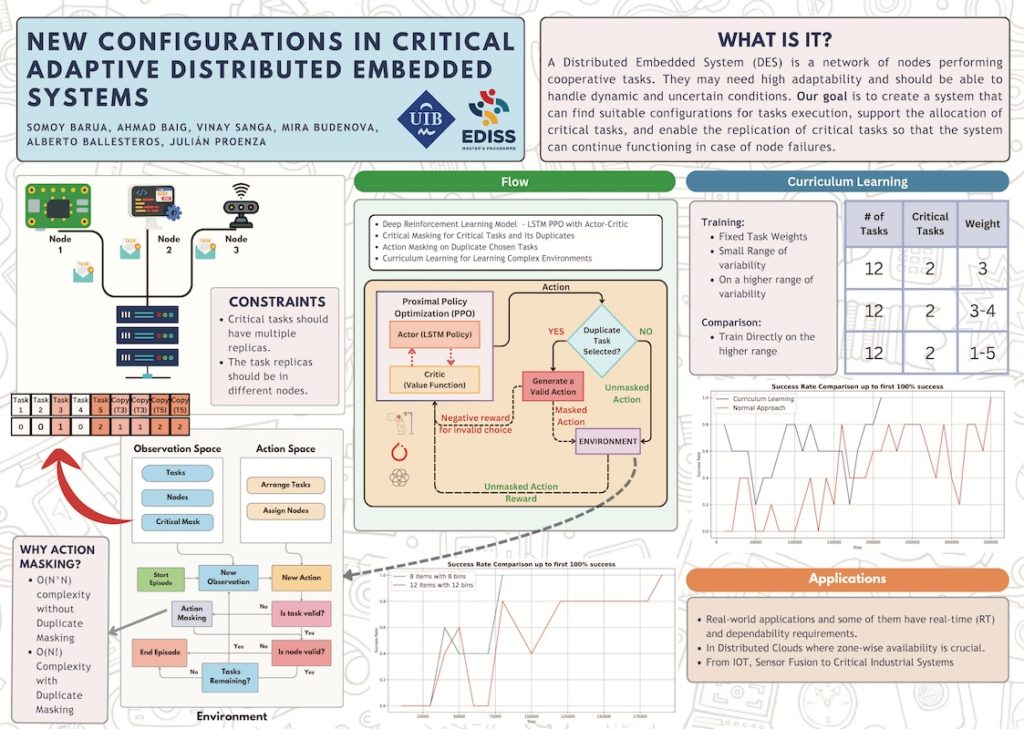
Team Arrhythmia
detection
Arup Sarkar, Minase Mengistu, Maheen Ghani, Naimur Rahman
Revolutionizing cardiac health, our research harnesses state of the art convolutional neural networks (CNNs) to detect Atrial Fibrillation, a common type of atrial arrhythmia, from remotely acquired ECGs.
By focusing on the nuanced differences between V1 and V2 leads and deploying our model on a Tensor Processing Unit, we achieve a impressive 97% detection rate.
This research not only advances cardiovascular diagnostics and promises significant societal impact by enabling early arrhythmia detection due to its asymptomatic nature but also leveraging Gradient Weight Class Activation Mapping (GRAD-CAM), we also ensure our findings are transparent and easily understood, making our model’s decision explainable and trustworthy.
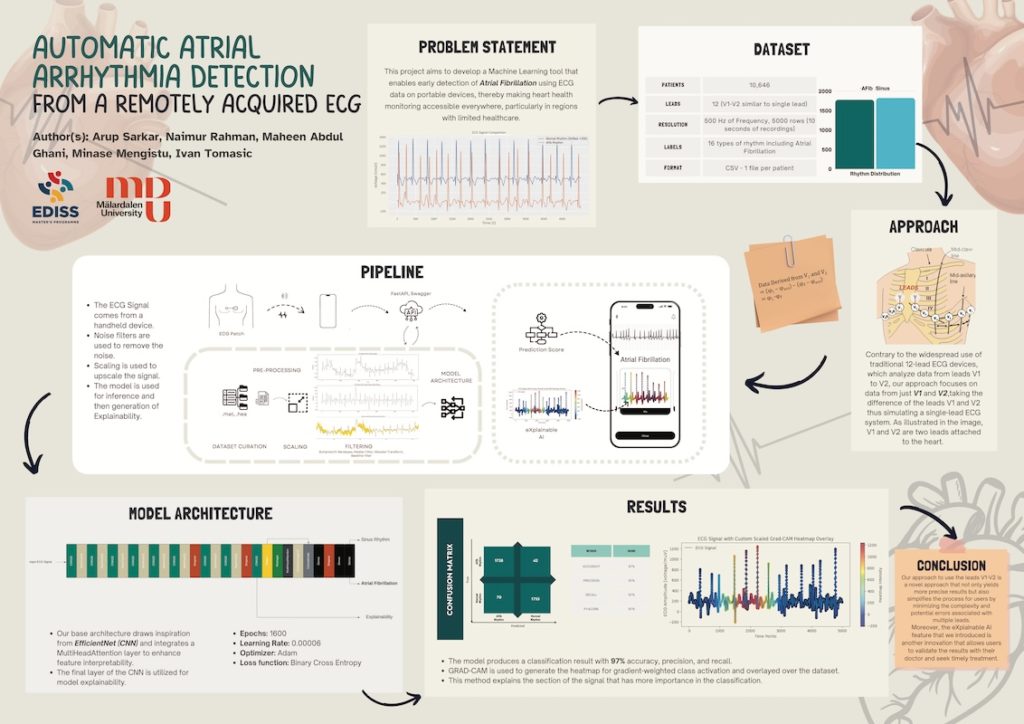
Last updated on 2 May 2024